In earlier posts I have discussed the reconstitution of manufacturing and infrastructure rebuilds in the United States. This will be a V-shaped turn its challenges is how to recruit in an environment of laborer scarcity. A gig economy platform will be used to source, qualify and manage the new workforce.
Recent jobs reports have been filled with interest factoids, there are now 9.93 million government workers than there are manufacturing workers. Many factors like labor cost differentials, regulations and taxes have forced manufacturing jobs to be sent overseas. Those manufacturing jobs have gone to the leisure and hospitality industry and those lower-paying leisure and hospitality jobs now outnumber manufacturing jobs. There are 3.3 million more leisure and hospitality workers than manufacturing workers.
Food services and drinking places (bartenders) employment is almost the same number of manufacturing jobs. And the number of government workers (22.213 million) is considerably larger than the number of food service and drinking place employees (11.384 million)
The market mechanism for manufacturing’s slow death was the structural reorganization that was necessary for manufactures to recover from the most recent recession. Plunging capital spending, weak exports hampered by a strong dollar and reduction in inventories. Soon we will see a huge lean manufacturing recovery, but how will those jobs be filled and who will fill them.
Employers often struggle to assess qualified applicants, particularly in contexts where they receive thousands of applications for job openings. Gig Economy platforms incorporate tools that offer algorithmic assessments of workforce data to identify variables that lead to stronger employee performance, resolve high employee attrition rates and flag the top applicants in a labor pool.
Data-driven employment is problematic for recruiting labor, its premise is that with the right calculation, the perfect employee can be found. This is particularly problematic in a labor market with high unemployment. Companies are leaving positions vacant for long periods of time as they search for candidates who fit a long list of micro-requirements, instead of opting to train employees or the easy hire or locally available labor for their jobs instead.
The use of hiring algorithms that rely on seemingly innocuous data to weed out higher-cost employees through proxies means that employers can use familiar scoring mechanisms to penalize or benefit job candidates, and do so by using rationales that constitute a black box of mysterious calculations to the job candidate
Companies do not intentionally engage in discriminatory hiring but their reliance on automated systems, algorithms, our current regulatory framework designed around a shorter tether between capital and labor is unprepared to address inequalities in data centric hiring systems.
If employers only look at applicants above a certain score threshold algorithms might blur opportunities through sorting mechanisms. Should applicants whose homes are far from their workplaces be scored lesser than the closer ones, what if this disparately impacts the members of disadvantaged socio-economic communities
Hiring algorithms are designed using historical data to create predictions about which qualities correlate to a strong job performance. It is unclear what employers are optimizing for in their attempts to maximize efficiencies and minimize risk and costs and there are barriers to transparency in algorithmic calculations, disclosing the calculations increases the probabilities that applicants will try to “game the system.
The End
23Feb
Algorithmic Recruiting of Laborers on a Gig platform
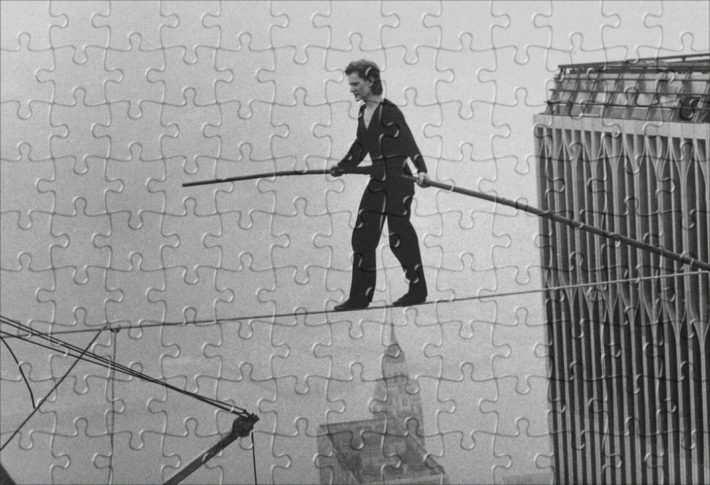